Topological phase transitions
Detecting topological phase transitions from measurement data is hard. Can neural networks help us understand these phase transitions better? The answer is yes: we built predictive models that can automatically find the boundaries of these exotic phases.
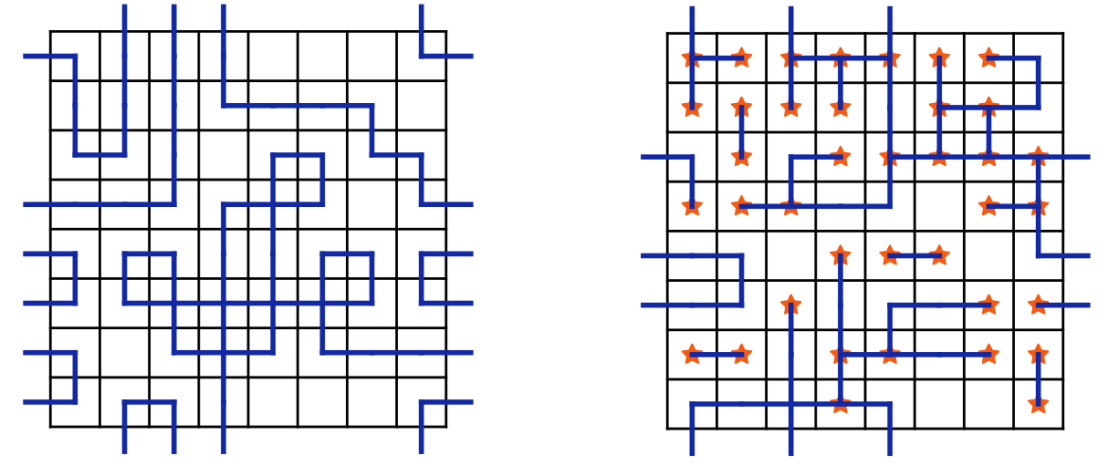
Identifying phase transitions is one of the key questions in theoretical and experimental condensed matter physics alike. Topological phase transitions represent an outstanding challenge, as they cannot be described by a local order parameter. In addition, tools developed to detect topological phase transitions as e.g. ground state degeneracy, topological entanglement entropy or the entanglement spectrum are not only demanding to compute but require knowledge that might not be experimentally accessible.
There is hence a strong demand to develop new probes to detect topological phase transitions. It is particularly useful to have access to a generic non-system specific probe, as e.g. the specific heat for thermal transitions.
In our work, we use a neural network based approach to detect phase transitions without local order parameter. The central idea of our approach is to distill a predictive model that relates input data from numerical or experimental studies to the output in the form of a known tuning parameter. We can then infer the position of the phase transition by analysing the network’s performance in predicting this parameter. We investigate two generic models hosting phase transitions without a local order parameter, the finite-temperature cross-over in Wegner’s Ising gauge theory and Kitaev’s toric code in the presence of disorder.
Reference
Greplova E, Valenti A, Boschung G, Schäfer F, Lörch N, Huber SD. Unsupervised identification of topological phase transitions using predictive models, external page New Journal of Physics 22, 045003 (2020)